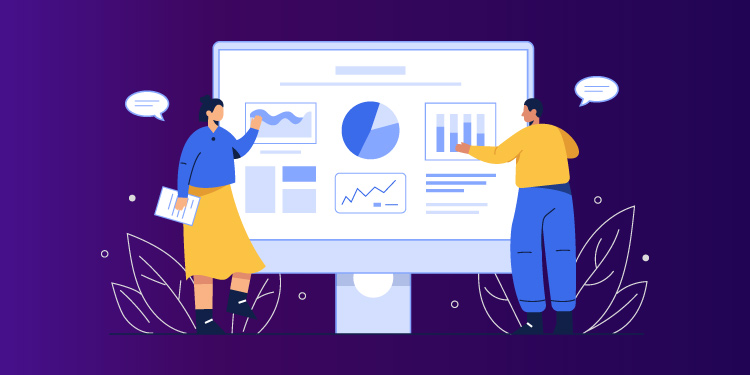
Data Enrichment Strategies to Drive Growth in 2022
Marshal, December 15, 2021
We all understand data enrichment at a high level. Before we dive deep into data enrichment strategies we need to know why we need data enrichment in the first place.
Data enrichment protects businesses against data deterioration caused by outdated and redundant entries. It allows them to increase the value and quality of their datasets while also gaining valuable insights. Data enrichment practices reduce the time and effort required to perform the work by allowing for smart automation.
Brands can use data enrichment to offer individualized services and improve the overall consumer experience. This not only improves customer satisfaction but also lowers churn. It can also save money by accessing data automatically, combining redundancies, and reducing errors while maintaining updated profiles.
How Does an Enterprise Stand to Benefit from Data Enrichment?
By providing access to more accurate data, data enrichment aids organizations in making better decisions. Data enrichment enhances the quality of your data, not simply the quantity. Here you not only add to the data points, you also double-check their accuracy. Validating and enhancing data can reveal new opportunities you may have otherwise overlooked!
Some of the Data Enrichment Strategies that will Help Drive Growth in 2022
1. Having a No Compromise and Continual Approach on Data Quality
The process of data enrichment is ongoing. As the needs of the customers change, it must be done frequently. Scraping internet data sources and gathering data from them can help businesses create a continual enrichment process. Inadequate data gathering may lead to incorrect conclusions, which consumers may not recognize for a long time. Due to erroneous insights, this might result in significant losses for businesses. In addition, data quality must be within accepted criteria and current.
Direct or personal data, internal data from CMS, ERP, digital services, or other production systems, or external third-party data can all be used in Data Enrichment procedures. Because the information is obtained directly from the clients, the direct source is often known as first-party data in consumer businesses.
Four easy steps can be taken to improve the quality:
- Profile data to find and explain irregularities after gaining access to the data sources.
- Establish data cleansing and standardization criteria to ensure that the data is fit for purpose. Completeness, conformance, consistency, accuracy, integrity, and duplication can all be used as guidelines.
- When it comes to data quality processes, follow the established guidelines. Then double-check the information.
- Data quality should be tracked and reported on at all times, for all goals, and across all business applications.
Additional Read: Top 6 result-critical benefits with Data Enrichment
2. Building a Streamlined Data Pipeline by Utilizing Top Notch Data Normalization and Transformation Techniques
Data is ready to be integrated with the current database after the data quality check and data cleaning. To ensure that all production systems have the most recent data you must employ extract, transform, and load operations on the datasets. The normalization and transformation procedure is divided into three stages:
- In the Extraction phase, data is extracted from an existing database.
- The data is then upgraded and translated into a more useful format during the Transformation phase.
- In the Loading phase, the data has been transformed and is ready to be used. It is now loaded into the spot where it is needed.
It’s vital to examine the data in your Data Lake and any other data repositories before moving on to the extraction step. You must also determine whether the given data requires further correction and whether any additional information is required to achieve the desired outcomes. Organizations will have access to vast amounts of data, but not all of it will be useful to their operations. As a result, data extraction, normalization and transformation techniques are used to ensure that data may be used for the intended purpose through Data Enrichment.
3. Removing Data Redundancies, Outliers, and Duplicates while Mapping Data Points for Accuracy
Another step in the Data Enrichment process is extrapolating data. Engineers can use fuzzy logic techniques to extract more information from a raw data source. It’s crucial to match data with existing duplicates after data cleansing. Probabilistic duplication is one of the most difficult to detect. Probabilistic duplicates are data fields that may represent the same thing yet differ in attributes such as spelling names, phone numbers, or even email addresses. Regular overall record matching is required to determine whether these data are reflecting the same entity. As a result, maintaining a high level of accuracy during data matching is essential. Higher precision indicates that the data is portraying the same thing.
Data deduplication should also be done at the same time. This is necessary because when an entity’s information is modified, there’s a potential that instead of updating the existing record, a new one will be created by accident. This is why, during the Data Deduping process, duplicates or superfluous data records must be eliminated. Data enrichment services can only be successful if duplicates are removed and records are cleaned.
4. Tagging and Segmenting Data Clusters to Enable Bespoke Messaging
It is always preferable to categorize or group data into distinct tags. Data enrichment best practices and activities will pay off more if organizations can narrow and “tighten” data sets to focus on certain target segments. They must first establish their target market and business objectives before segmenting. Then they can assess which procedures will be supported by the improved data to meet contextually relavant messaging and campaign objectives. Finally, after segmentation, companies must establish a data flow between all of their tools. This is where data becomes a useful resource.
5. Time-Bound Monitoring and Enhancement of Data Repositories
Companies must run Data Enrichment regularly. Any information on an entity is subject to change over time. Using out-of-date information might stymie corporate operations. The buying habits of customers are always changing. As a result, data should be regulated such that it is cleaned and validated regularly to assure consistent and continuous data quality in the future. In the long run, data matching and deduping assist in achieving this accuracy.
Data monitoring is another approach to accomplish this. Setting up controls to ensure that data is compliant with data quality and business requirements is part of data monitoring. Businesses may also purchase access to other databases while updating data to look for additional information on their clients and consumers, which they can then add to their database.
Additional Read: Relevant? Unique? Enough? Understanding the need for data enrichment and net new data
6. Leveraging AI and Machine Learning Models
Data enrichment techniques essentially involve combining two datasets with similar characteristics, like customer lists and purchase histories. For example, data enrichment has become increasingly important as businesses are using machine learning algorithms more often to analyze their customers’ behavior or predict future behaviors based on past actions. Data is being collected from various sources to better understand customer behavior. The use of AI and Machine Learning for data enrichment is becoming more popular as it can help marketers create a custom experience for customers that are tailored to their needs. It also ensures that marketing messages are sent at the right time with the right message so customers see what they want when they need it most!
AI and Machine Learning are great for data enrichment. They can do things like clean out duplicates, find typos, or find companies that you might not have thought to include in your search. But what if the data is too messy? We all know how hard it can be to sift through piles of files, social media posts, and surveys — we need help! Luckily there’s a way: using AI and ML with human-in-the-loop approaches will allow you to get the most accurate results possible. AI and ML work best when they’re used as tools by people who understand their strengths and limitations. You should avoid relying on them exclusively because their algorithms may result in biased outcomes or fail altogether due to unanticipated circumstances.
In Conclusion
As the year progresses, it’s time to start thinking about how you’ll grow your business in 2022. Data enrichment strategies are one of many ways that can lead to increased growth and profitability for any company looking to take its digital marketing game up a notch.
At Lake B2B, we’ve helped countless clients use data-driven methods like customer journey mapping or predictive analytics with success rates as high as $1 million+ over six months! If these sound interesting but out of reach right now, don’t worry–we’re here every step of the way to help make sure you get started on this path down the road.
Contact us today if you want more information on how our team could be the key ingredient that unlocks your next level.